Google has continued to develop its medical question-answering language model, Med-PaLM, which was first introduced in December of 2021. Med-PaLM was created by combining a special soft prompting method with responses from four clinicians to produce a model that can answer medical questions at an expert level. The language model was able to perform similarly to human experts in most of the benchmarks tested and produced potentially harmful responses only slightly more often than human experts.
Med-PaLM was also able to potentially pass the U.S. Medical Licensing Examination, achieving 67.2 percent correct when tested with licensing-style questions, compared to the required 60 percent. The model was capable of answering both multiple-choice and open-ended questions and reasoning about its responses.
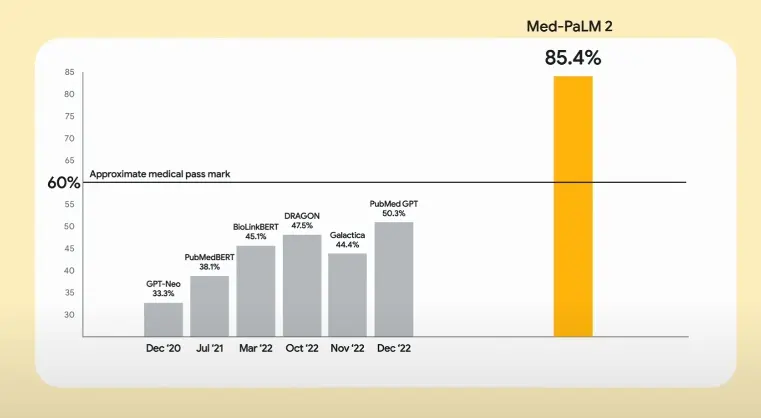
The latest version of Med-PaLM, Med-PaLM 2, is even more accurate than its predecessor. It can answer medical exam questions at an “expert doctor level” with an accuracy rate of 85 percent. This is an 18 percent increase in performance over the previous version, making it significantly more accurate than comparable language models in medical tasks.
However, there are still significant gaps in Med-PaLM 2’s ability to answer medical questions, according to the team. The language model was tested against 14 criteria, including scientific factuality, accuracy, medical consensus, reasoning, bias, and harm, and there were notable gaps in its performance. The team is working to address these gaps and improve the language model’s ability to answer medical questions.

The team’s research shows that language models like Med-PaLM have the potential to change the healthcare industry. By accurately answering medical questions, these models could help doctors and other healthcare professionals make more informed decisions and improve patient outcomes. Additionally, they could potentially reduce healthcare costs by streamlining the process of answering medical questions and providing patients with more accurate information.
Despite the promise of these language models, there are concerns about their potential to produce harmful or incorrect responses. The team found that Med-PaLM produced potentially harmful responses 5.9 percent of the time, compared to 5.7 percent for human experts. While the difference is small, it highlights the need for continued research and development to ensure that these models meet Google’s quality standards and are safe for use in medical settings.